Toward Food Security in Africa: Regenerative Agriculture Needs AI for Precision
We urgently need to tackle the challenge of food security in Africa in the face of climate change and conflicts; adopt sustainable and regenerative agricultural production to preserve soil health for future generations; and embrace Precision Agriculture practices to increase yields for our hard working farmers.
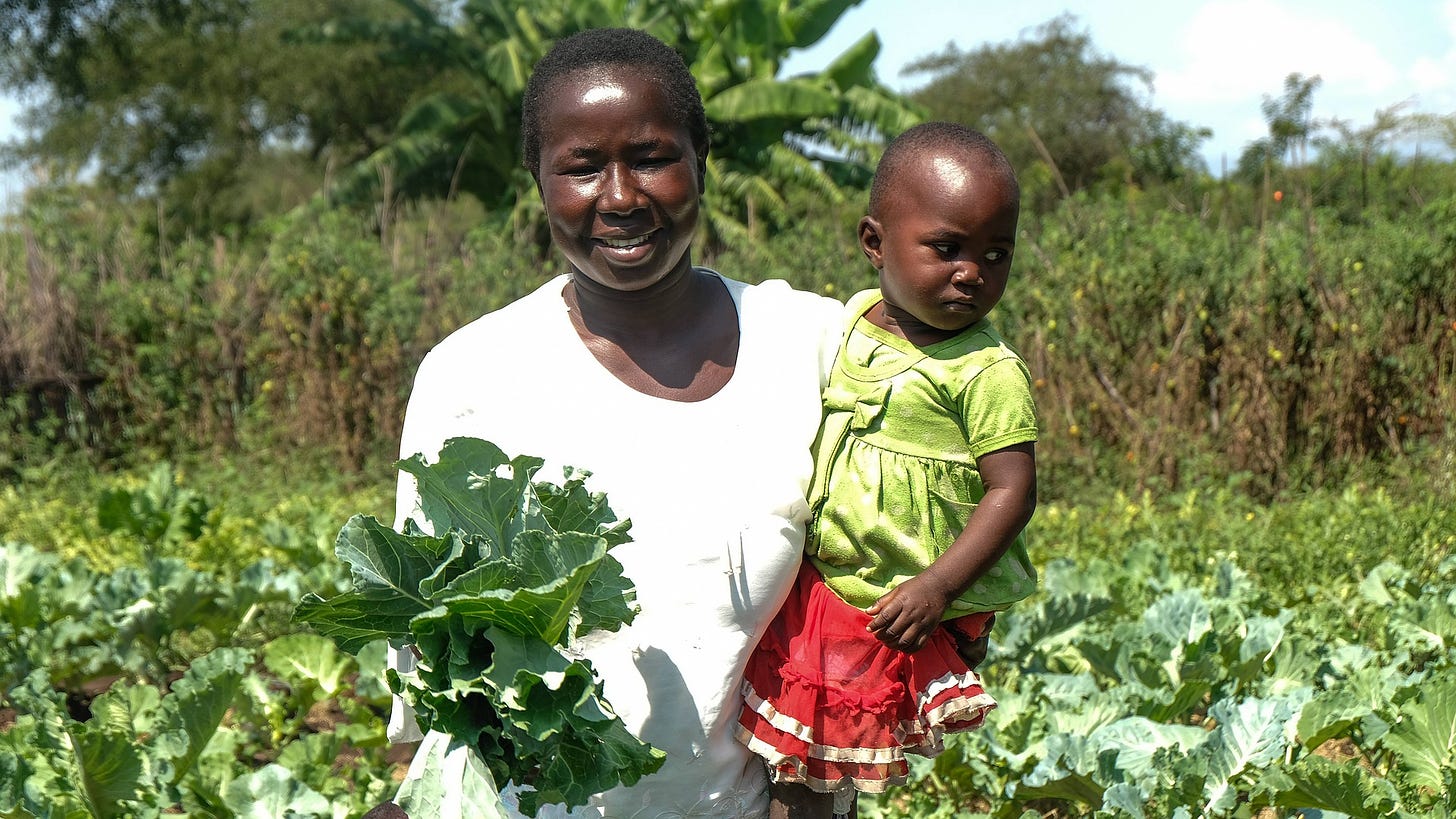
The ongoing conflict between Russia and Ukraine has revealed the danger of depending on the importation of food and fertilizers [11]. African indigenous food not only tastes good but is also nutritious and can contribute to good population health. As Hippocrates once remarked, “Let thy food be thy medicine and medicine be thy food.” In fact, there is growing evidence that the vitamins and nutrients in modern food have decreased over the last 70 years [14].
Re-introducing drought-resilient and highly nutritious African indigenous food (so called “forgotten food crops”) will be essential to ensure food security in the face of climate change and rising temperatures, particularly in areas like the Sahel region of Africa. According to a paper titled Forgotten food crops in sub-Saharan Africa for healthy diets in a changing climate by van Zonneveld et al. [18]:
“Projecting forward to the year 2070, we show that a prioritized collection of these [forgotten] crops, differentiated by food groups, has high potential to diversify cropping systems of major staples to support climate-resilience and nutrition in the [sub-Saharan Africa] SSA region.”
In addition to re-introducing “forgotten food crops” to ensure food security, farmers in Africa should also embrace Regenerative Agriculture practices. Comparison of conventional and regenerative farming practices demonstrates the advantages of the latter on soil health and the nutrient density of crops and livestock [15].
At Atlantic AI Labs, we take a complex system approach to agriculture by fully embracing the vision of One Health which promotes a unified approach to the health of people, animals, and the environment.
Current challenges to food security in Africa include the following:
Intensive monoculture.
Use of imported agrochemical inputs like fertilizers and pesticides leading to soil degradation, loss of biodiversity, and human health issues like cancer.
Water scarcity and drought exacerbated by climate change.
Limited availability of resources including land, labor, financing, and agricultural equipment.
Plant diseases, pests, and weed.
The health and production of livestock which is related to the health of people and the environment. Climate change and drought have also caused an increase in traditional pastoral herd mobility toward greener pastures and violence between farmers and herders.
AI and robotics can serve as catalyst for agriculture modernization in Africa. Applications of AI in agriculture includes using advanced sensors to collect farm data and imagery for Machine Learning-driven decision making. Robotics applications include the use of uncrewed aerial (UAV) and ground vehicles (UGV).
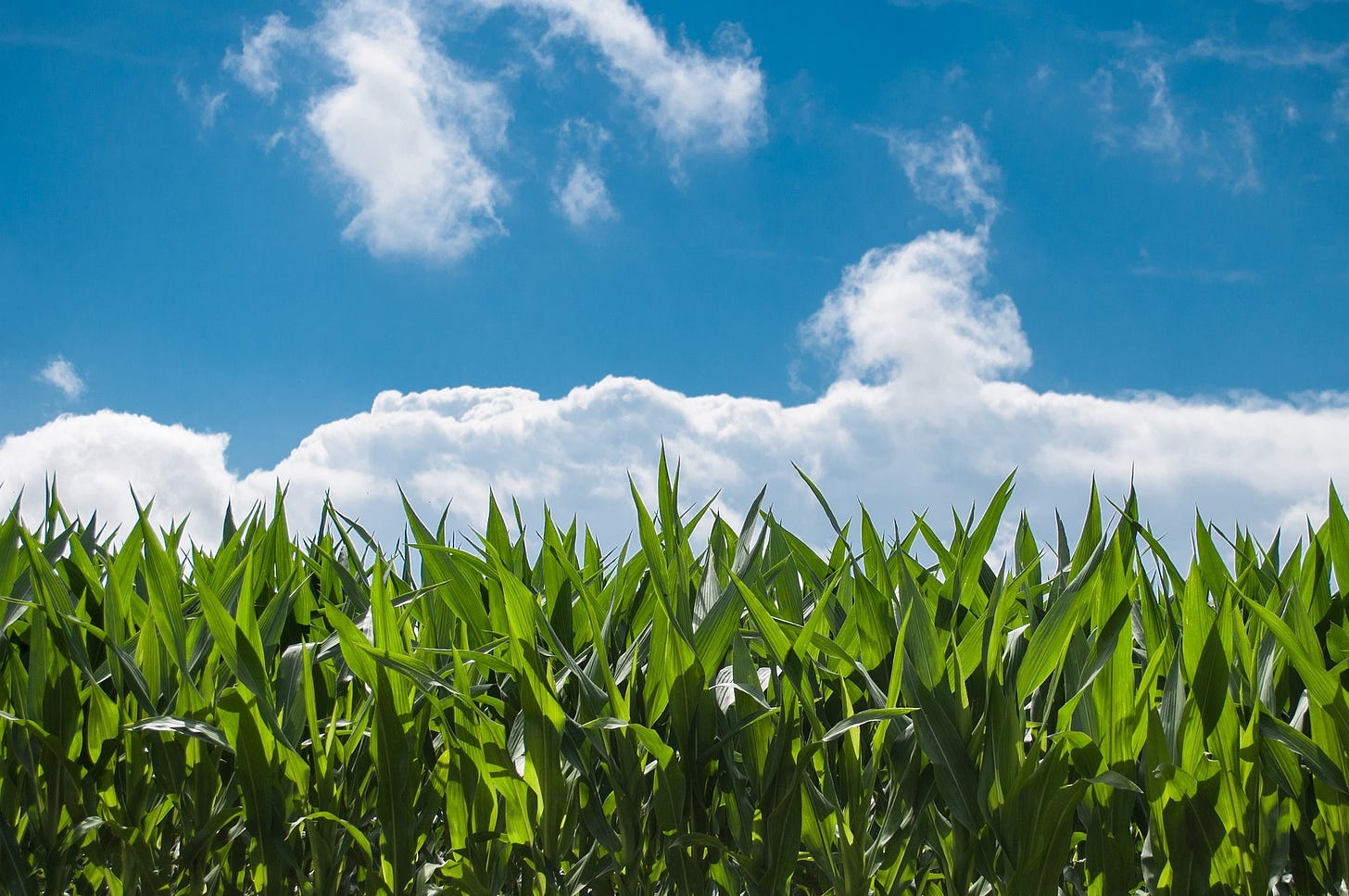
Evidence-Based Regenerative Agriculture
Regenerative Agriculture practices aim to:
Maintain or restore soil health and decrease soil erosion.
Increase microbial and other species diversity.
Improve soil moisture retention to increase resilience to droughts or flooding during high rain fall.
Improve soil carbon sequestration.
Improve yields in a sustainable manner.
Regenerative practices include:
Crop diversification through agroforestry, intercropping, crop rotations, cover crops.
Organic fertilizers like manure, mulching, biochar, and residue and waste retention.
Low or no tillage solutions.
Natural pest control and integrated pest management.
Cultivar mixtures.
Low or no chemical inputs like synthetic fertilizers and pesticides.
Use of sustainable energy like solar-powered irrigation pumps.
Integrating of crops and livestock through rotational light grazing or a technique known as adaptive multi-paddock grazing (AMP) [13].
Drought, insect, and disease resistant crop seed [17, 18] and soil management practices [16].
A report titled Aligning regenerative agricultural practices with outcomes to deliver for people, nature and climate [1] by The Food and Land Use Coalition (FOLU) provides an evidence-based approach to evaluating regenerative agriculture practices. The goal is to track relevant metrics to ensure that regenerative practices deliver the right outcomes in a local context.
Collecting Data with Sensors
Machine Learning requires a lot of data for accurate predictive modelling.
Affordable Internet of Things (IoT) devices can be used to collect, aggregate, and analyze large and diverse data sets on the weather (e.g., air temperature, humidity, radiation, and precipitation), location, soil health, seeds, crops, etc. Biometric sensors can be used to collect data on the health and behavior of animals.
Precision Agriculture practices like variable rate irrigation (VRI) [6] and variable rate nitrogen (VRN) fertilization [7,8,9,10] are made possible thanks to advances in telemetry, hyperspectral imaging, and aerial and satellite-based remote sensing.
Hyperspectral and multispectral sensing [12] has been used for yield prediction, estimating nitrogen content, disease detection, estimation of evapotranspiration, and crop water stress for precision irrigation.
Machine Learning
Advances in machine vision using Deep Learning can be leveraged for tasks such as monitoring, detection, semantic segmentation, and classification. While Deep Learning is effective for imaging applications like disease detection, other methods like Random Forests and Extreme Gradient Boosting (XGBoost) should also be considered for time series and tabular data analysis [5].
A comprehensive review [2] identified the following categories of Machine Learning (ML) applications in Agriculture:
Crop Management
Water Management
Soil Management
Livestock Management.
Crop Management applications were further subdivided as follows:
Yield Prediction
Disease Detection
Weed Detection
Crop Recognition
Crop Quality.
Robotics
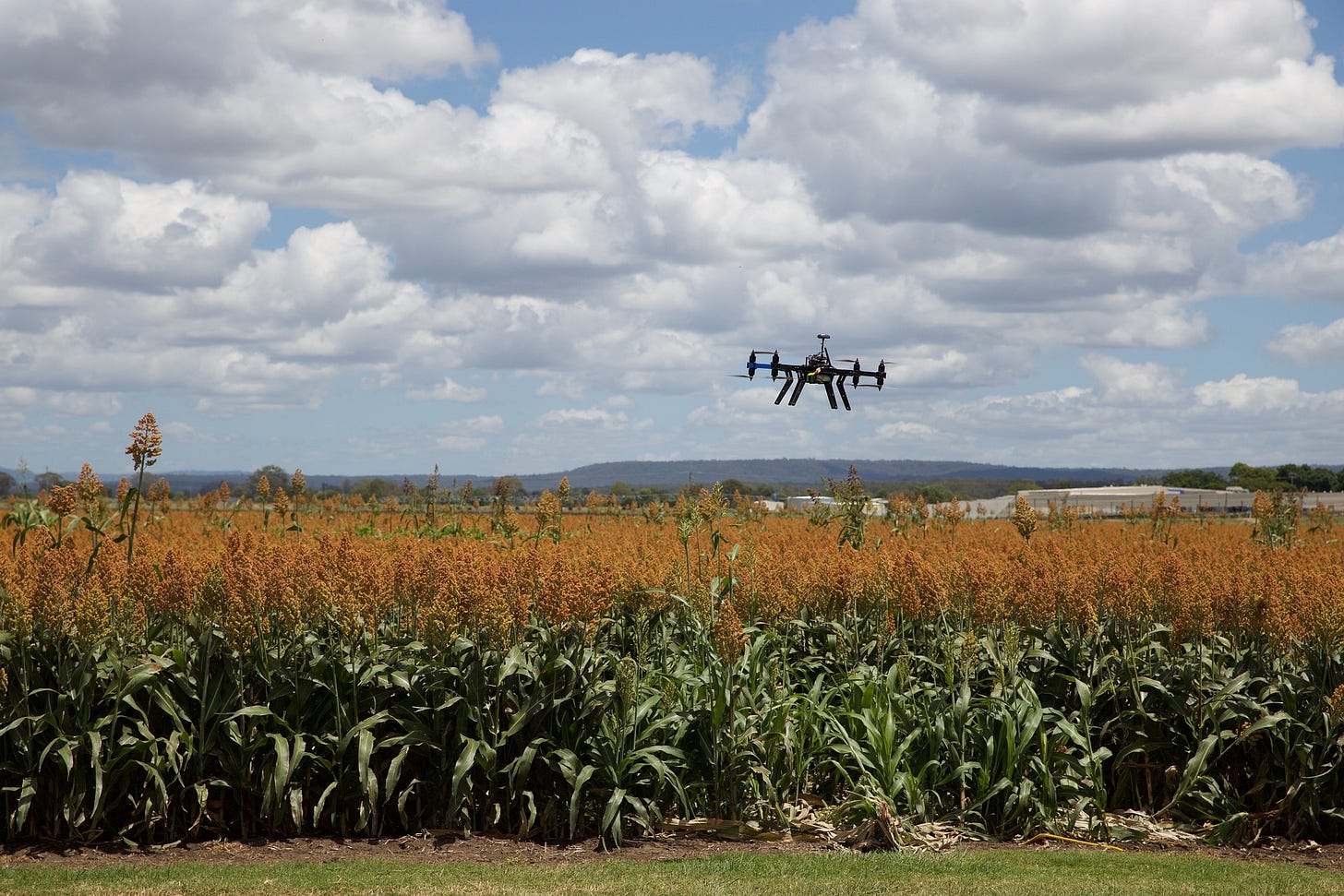
Robotic applications include uncrewed aerial (UAV) and ground vehicles (UGV) equipped with advanced sensors, actuators, and manipulators for soft robotic grasping. Sensor data fusion from GPS, INS, LiDAR, and vision cameras allows simultaneous localization and mapping (SLAM) for precise navigation with centimeters accuracy.
For example, a drone can fly over large areas of farm land in few minutes and send imagery in real-time to a UGV [3] which can make precise decisions and targeted interventions (e.g., pest or weed control, irrigation, etc.) while reducing waste.
Robots [4] are useful throughout the agricultural production life cycle including:
Micro-tillage — a low-tillage solution as opposed to intensive ploughing
Seed placement and mapping
Fertilizing
Irrigation
Weeding (removing unwanted plants)
Monitoring
Harvesting
Transportation.
References
[1] The Food and Land Use Coalition (FOLU), Aligning regenerative agricultural practices with outcomes to deliver for people, nature and climate https://www.foodandlandusecoalition.org/knowledge-hub/regenag-people-nature-climate/
[2] Liakos, Konstantinos G., Patrizia Busato, Dimitrios Moshou, Simon Pearson, and Dionysis Bochtis. "Machine learning in agriculture: A review." Sensors 18, no. 8 (2018): 2674. https://www.mdpi.com/1424-8220/18/8/2674
[3] Pretto, Alberto, Stéphanie Aravecchia, Wolfram Burgard, Nived Chebrolu, Christian Dornhege, Tillmann Falck, Freya Fleckenstein et al. "Building an aerial–ground robotics system for precision farming: an adaptable solution." IEEE Robotics & Automation Magazine 28, no. 3 (2020): 29-49. https://ieeexplore.ieee.org/abstract/document/9177181
[4] Duckett, Tom, Simon Pearson, Simon Blackmore, Bruce Grieve, Wen-Hua Chen, Grzegorz Cielniak, Jason Cleaversmith et al. "Agricultural robotics: the future of robotic agriculture." arXiv preprint arXiv:1806.06762 (2018). https://arxiv.org/ftp/arxiv/papers/1806/1806.06762.pdf
[5] Grinsztajn, L., Oyallon, E. & Varoquaux, G. Why do tree-based models still outperform deep learning on tabular data? arXiv:2207.08815 (2022).
[6] O’Shaughnessy, Susan A., Steven R. Evett, Paul D. Colaizzi, Manuel A. Andrade, Thomas H. Marek, Derek M. Heeren, Freddie R. Lamm, and Jacob L. LaRue. "Identifying advantages and disadvantages of variable rate irrigation: An updated review." Applied Engineering in Agriculture 35, no. 6 (2019): 837-852.
[7] Zhang, Jiayi, Weikang Wang, Brian Krienke, Qiang Cao, Yan Zhu, Weixing Cao, and Xiaojun Liu. "In-season variable rate nitrogen recommendation for wheat precision production supported by fixed-wing UAV imagery." Precision Agriculture 23, no. 3 (2022): 830-853.
[8] Heiß, Andreas, Dimitrios S. Paraforos, Galibjon M. Sharipov, and Hans W. Griepentrog. "Real-time control for multi-parametric data fusion and dynamic offset optimization in sensor-based variable rate nitrogen application." Computers and Electronics in Agriculture 196 (2022): 106893.
[9] Stansell, Jackson S., Joe D. Luck, Tyler G. Smith, Hongfeng Yu, Daran R. Rudnick, and Brian T. Krienke. "Leveraging multispectral imagery for fertigation timing recommendations through the N-time automated decision support system." In Autonomous Air and Ground Sensing Systems for Agricultural Optimization and Phenotyping VII, vol. 12114, p. 1211402. SPIE, 2022.
[10] Stansell, Jackson S., J. D. Luck, Tyler G. Smith, Hongfeng Yu, Daran R. Rudnick, and Brian T. Krienke. "N-Time: An Automated Decision Support System for Sensor-Based Fertigation Management." VDI Berichte 2395 (2022): 259-264.
[11] African Union leader Macky Sall to meet Putin on Friday to 'free cereal stocks' https://www.politico.eu/article/african-union-leader-macky-sall-meet-putin-friday-free-cereal-stocks/
[12] Adão, Telmo, Jonáš Hruška, Luís Pádua, José Bessa, Emanuel Peres, Raul Morais, and Joaquim Joao Sousa. "Hyperspectral imaging: A review on UAV-based sensors, data processing and applications for agriculture and forestry." Remote sensing 9, no. 11 (2017): 1110.
[13] Mosier, Samantha, Steve Apfelbaum, Peter Byck, Jim Ippolito, and M. Francesca Cotrufo. "Improvements in soil properties under adaptive multipaddock grazing relative to conventional grazing." Agronomy Journal 114, no. 4 (2022): 2584-2597.
[14] Stacey Colino, "Fruits and vegetables are less nutritious than they used to be" National Geographic, May 2, 2022 https://www.nationalgeographic.co.uk/environment-and-conservation/2022/05/fruits-and-vegetables-are-less-nutritious-than-they-used-to-be
[15] Montgomery, David R., Anne Biklé, Ray Archuleta, Paul Brown, and Jazmin Jordan. "Soil health and nutrient density: preliminary comparison of regenerative and conventional farming." PeerJ 10 (2022): e12848.
[16] Heitman, Joshua L., Dilia Kool, and Henrique DR Carvalho. "Soil management considerations for water resiliency in a changing climate." Agronomy Journal 115, no. 5 (2023): 2127-2139.
[17] Porch, Timothy G., Juan Carlos Rosas, Karen Cichy, Graciela Godoy Lutz, Iveth Rodriguez, Raphael W. Colbert, Gasner Demosthene, Juan Carlos Hernández, Donna M. Winham, and James S. Beaver. "Release of tepary bean cultivar ‘USDA Fortuna’with improved disease and insect resistance, seed size, and culinary quality." Journal of Plant Registrations (2023).
[18] van Zonneveld, Maarten, Roeland Kindt, Stepha McMullin, Enoch G. Achigan-Dako, Sognigbé N’Danikou, Wei-hsun Hsieh, Yann-rong Lin, and Ian K. Dawson. "Forgotten food crops in sub-Saharan Africa for healthy diets in a changing climate." Proceedings of the National Academy of Sciences 120, no. 14 (2023): e2205794120.