Introducing Evolving Engineering for Learning (EEL) toward Safe AI
March 4 was World Engineering Day and I had the opportunity to introduce EEL (Evolving Engineering for Learning) which is Atlantic AI Labs’ framework toward AI trustworthiness and safety. EEL is inspired by the aviation concept of “airworthiness” which has made air travel the safest mode of transportation by far. Just good old engineering evolving to support learning-enabled, autonomous, adaptive, high-dimensional, highly non-linear, partially observable, and non-deterministic behavior under uncertainties.
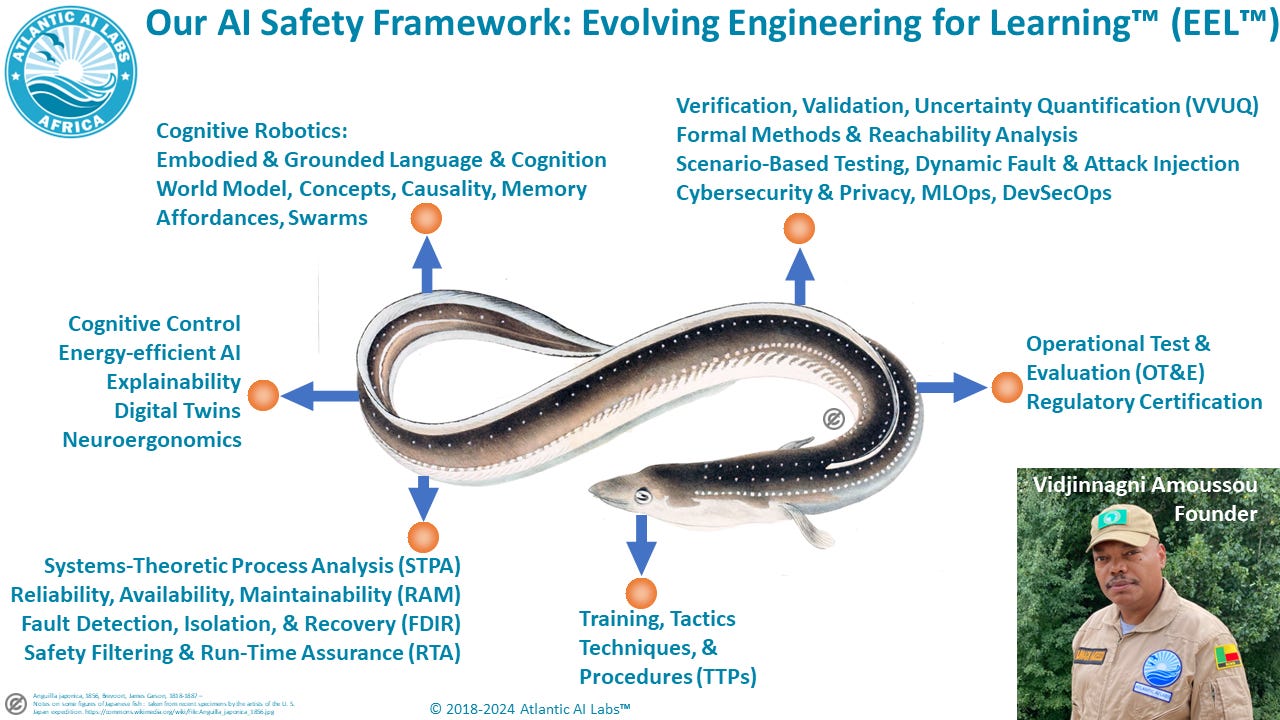
Please read key principles of the framework from the tail to the head of the eel in the slide — a whole-body approach so to speak. The eel is a fascinating sea creature and an inspiration (more on that later).
EEL is a framework for thinking and working responsibly toward Safe AI. It is not a product and it is domain-agnostic. For example, in the military aviation domain, the term Operational Test and Evaluation (OT&E) is used; while in medicine, prospective studies or Randomized Control Trials (RCTs) are used to evaluate the efficacy of AI interventions on patient-centered outcomes and generate evidence for regulatory approval.
According to Annex 8 to the Convention on International Civil Aviation:
“The application of airworthiness defines the condition of an aircraft and supplies the basis for judgment of the suitability for flight of that aircraft, in that it has been designed with engineering rigor, constructed, maintained and is expected to be operated to approved standards and limitations, by competent and approved individuals, who are acting as members of an approved organization and whose work is both certified as correct and accepted on behalf of the State. The airworthiness of aircraft ranges thus from the initial approval of a new aircraft design to ensuring an aircraft’s on-going safety standards.”
Generative AI that “hallucinates” is all the rage these days but can be problematic in certain safety-critical domains and applications. The term “hallucination” is being used to describe the tendency of large language models (LLMs) to make things up. The more I read about the concept of “hallucination” in the neuroscience and the cognitive sciences literature, the less convinced I am that it is appropriate for describing the behavior of LLMs. Use of the term “hallucination” to describe LLMs making things up is clearly a case of anthropomorphism which does not help in elucidating their true inner workings. However, neuroscience is itself not immune to anthropomorphism. That is the subject of another post.
In fact, we submit that this tendency to make things up is not a bug, but feature of LLMs. This is reminiscent of the famous paper Adversarial Examples Are Not Bugs, They Are Features by Ilyas et al.
Lawyers from a New York law firm who submitted fake cases generated by ChatGPT have been sanctioned by a judge who said ethics rules “impose a gatekeeping role on attorneys to ensure the accuracy of their filings.” The law firm stated: “We made a good faith mistake in failing to believe that a piece of technology could be making up cases out of whole cloth.” Users and designers of ChatGPT have agency; ChatGPT does not.
The argument could be made that as statistical next-token predictors in Internet-scale text corpora, autoregressive LLMs are a dead-end toward safe AI which requires factuality, causality, reasoning, and planning.
From a safety, privacy, legal, and regulatory standpoint, we believe that so called Foundation Models (e.g., DALL-E, GPT-4) are on shaky ground. Investigations by regulatory bodies are underway in a number of countries. In the US, the Federal Trade Commission (FTC) has launched an investigation into OpenAI over consumer protection matters. There are ongoing legal cases and class action lawsuits against certain companies that have released Generative AI products for the unauthorized mass collection of online content posted by internet users and commercial publishers.
In the “Risk Factors” section of Microsoft’s SEC filing for the quarter ended March 31, 2023, one can read:
“AI algorithms or training methodologies may be flawed. …As a result of these and other challenges associated with innovative technologies, our implementation of AI systems could subject us to competitive harm, regulatory action, legal liability, including under new proposed legislation regulating AI in jurisdictions such as the European Union (‘EU’), new applications of existing data protection, privacy, intellectual property, and other laws, and brand or reputational harm.”
In a paper titled Large language model AI chatbots require approval as medical devices published in the journal Nature Medicine in June 2023, the authors write:
“Chatbots powered by artificial intelligence used in patient care are regulated as medical devices, but their unreliability precludes approval as such.”
The growing list of companies that have banned or restricted access to ChatGPT for their employees at work includes:
Spotify
Northrop Grumman
Apple
JPMorgan Chase
Verizon
iHeartRadio
Citigroup
Samsung
Goldman Sachs
Wells Fargo
Bank of America
Deutsche Bank
Amazon.
Cognitive Robotics provides a framework for truly grounding both language and cognition in sensorimotor interactions with the physical environment (the umwelt) and social interactions with people for learning socio-cultural knowledge and norms. The embodied and enactive accounts of language and cognition are well-established in neuroscience and the cognitive sciences.
Tackling the complex subject of intelligence will necessitate an interdisciplinary approach to draw on diverse bodies of knowledge for creating a unified framework. As the physicist Richard Feynman explained:
“If our small minds, for some convenience, divide this universe, into parts — physics, biology, geology, astronomy, psychology, and so on — remember that nature does not know it!”
We are not suggesting that Cognitive Robotics is an easy path to AGI. A friend who works in the automotive industry told me that achieving Level 5 autonomous driving is as hard as sending humans to Mars and back. An article by Kirsten Korosec published in Bloomberg on October 26, 2022 and titled Even After $100 Billion, Self-Driving Cars Are Going Nowhere was a much needed reality check.
After Cruise shut down its robotaxi operations in the US, an article published in the New York Times on November 3rd, 2023 titled, G.M.’s Cruise Moved Fast in the Driverless Race. It Got Ugly states:
“Those vehicles were supported by a vast operations staff, with 1.5 workers per vehicle. The workers intervened to assist the company’s vehicles every 2.5 to five miles, according to two people familiar with its operations.”
US president John F. Kennedy once remarked:
“We choose to go to the Moon in this decade and do the other things, not because they are easy, but because they are hard; because that goal will serve to organize and measure the best of our energies and skills, because that challenge is one that we are willing to accept, one we are unwilling to postpone, and one we intend to win, and the others, too.”
What we’re learning from the on-going conflict between Russia and Ukraine is that victory is being decided not in cyberspace and not even in airspace (since neither side can gain air superiority at the time of writing), but in muddy World War 1-style trenches in the Eastern Donbas region of Ukraine. To defend freedom and democracy, a multi-domain approach will certainly be required, but the physical battlespace is where victory is ultimately achieved. So our AI-enabled defense systems will need to operate effectively in the physical environment, not just in a chat box.
We can make our planet a better place by providing food security, healthcare, and education to the most vulnerable populations in the world. It is the reason why Atlantic AI Labs also works in applying AI responsibly to tackle these challenges.
We believe that embodiment can help address key ingredients that are often missing in today’s AI systems: a World Model, causal reasoning, concept learning, affordances, and memory. In particular, from the perspective of embodied cognition, human conceptual processing activates multimodal sensorimotor simulations. Evidence suggests that the execution of an action and the off-line mental simulation of that action recruit the same neural substrate.
Safe AI requires factuality. But how are facts learned, encoded in memory, and recalled? Beyond their role in forming a Cognitive Map for spatial navigation (an “inner GPS” so to speak), place cells and grid cells in the hippocampal-entorhinal system also support the encoding and organization of other non-spatial and abstract information.
Conceptualization and meaning are grounded in the agent’s sensorimotor experiences. These experiences are stored in episodic memory which is necessary for life-long learning, decision making, and planning. The concepts and facts learned through these experiences and their meaning are stored in semantic memory independently of their spatiotemporal context.
Concept learning starts early in infancy — a research topic in the field of developmental psychology — and continues throughout the adult lifespan. It is the foundation of human imagination and the ability for mental simulation and also the key to creativity and innovation. Compositionality and concept blending endow humans with the ability to compose new arbitrary concepts from existing ones. For example, one can imagine the concept of a hybrid creature of human, bird, and lion which does not exist in the real world. Conceptual metaphors are pervasive in human language, discourse, and even common-sense reasoning and represent cross-domain mapping of concepts. Examples of grounded metaphors are: “to beat a dead horse” or “a roller coaster of emotions.”
By interacting with the umwelt through embodiment, the agent also learns “affordances” — a concept proposed by psychologist James Gibson in 1966 to describe the actions that an object or agent in the environment affords its observers (a fundamental aspect of common-sense reasoning). A self-driving car needs to learn affordances for safety. For example, it is not ok to crash into emergency vehicles and parked police cars.
Metacognition allows agents to monitor the accuracy and uncertainty of their perception of the environment and to assess and regulate their own reasoning and decision-making processes. Conformal Prediction is a method that can produce uncertainty sets or intervals of the model's predictions with guarantees based on probabilities that can be specified by the user.
Pure control approaches to autonomy rely on techniques like Deep Reinforcement Learning (DRL) and Model Predictive Control (MPC). To achieve safe Level 5 autonomy, we argue that these approaches are insufficient and must be augmented and integrated within a Cognitive Architecture — an approach that we call Cognitive Control, a concept borrowed from neuroscience and the cognitive sciences.
A Cognitive Architecture implements a computational model of various mechanisms and processes involved in cognition such as: perception, memory, attention, concept learning, affordances, causality, reasoning, decision making, planning, motor control, language, imagination, social interaction, self-awareness, theory of mind (ToM), metacognition, and consciousness (yes, the “c-word” which we believe should also be brought into the realm of scientific inquiry).
These human cognitive abilities came in handy when on January 15, 2009, Captain Chesley “Sully” Sullenberger made the quick decision to ditch the Airbus A320 of US Airways flight 1549 on the Hudson River after the airplane experienced a complete loss of thrust in both engines due to the ingestion of large birds. All 150 passengers and 5 crewmembers survived. Simulation flights were conducted as part of the US National Transportation Safety Board (NTSB) investigation. In the final accident report, the NTSB concluded:
“The captain's decision to ditch on the Hudson River rather than attempting to land at an airport provided the highest probability that the accident would be survivable.”
This event provides a good case study for autonomy research about decision making under uncertainty.
In the paper Attention to action: Willed and automatic control of behavior, Cognitive Scientists Don Norman and Tim Shallice suggest the following types of tasks for which attentional Cognitive Control is necessary:
“They involve planning or decision making
They involve components of troubleshooting
They are ill-learned or contain novel sequences of actions
They are judged to be dangerous or technically difficult
They require the overcoming of a strong; habitual response or resisting temptation.”
NeuroSymbolic AI is being touted as a solution to the shortcomings of pure Deep Learning architectures for building robust general intelligence. Debates on the role of symbols in AI are not new and still flare up today on social media between AI researchers. What is the evidence from neuroscience and the cognitive sciences? Stanislas Dehaene is a cognitive neuroscientist who has written about the role of symbols in human cognition in domains like language, mathematics, or music and their neural substrate. According to Dehaene, these recursive and hierarchical tree structures evolved from neural circuits involved in spatial navigation and the representation of geometric shapes as can be found on prehistoric engravings in the caves. Symbols also a play a role in compressing information for memory storage. In a recent paper titled Symbols and mental programs: a hypothesis about human singularity, Dehaene et al. write:
“Why are humans the only species to have developed not only spoken and written languages, but also the languages of science, music, mathematics, visual patterns…? We argue that behind each of these domains of competence lies a specifically human mode of mental representation: discrete symbols and their composition in a recursive language [of thought] allow our species to build arbitrarily complex mental structures out of a very small set of initial primitives.”
Without advocating for a return to logic-based expert systems of the 1980s (also known as GOFAI or Good Old-Fashioned Artificial Intelligence), we believe that progress in AI should build on these previous efforts. The symbol grounding problem (SGP) can be addressed effectively in hybrid AI architectures comprising both symbolic and sub-symbolic representations through the autonomous agent’s embodiment and sensorimotor interaction with the environment. Two promising directions of research also leading to neuromorphic computing and energy-efficient AI are Hyperdimensional Computing aka Vector Symbolic Architectures (VSA) and the Assembly Calculus introduced in the paper Brain computation by assemblies of neurons by Papadimitrioua et al.
Human Factors engineering, Neuroergonomics, and AI Explainability are sine qua non for AI trustworthiness and safety. After an accident, the first question the public asks is: “what happened” and “why”. The aviation industry implements lessons learned from detailed accident investigations and reports and that has contributed to significant improvements in aviation safety.
Digital Twins enabled by Modeling and Simulation (M&S) will play a key role throughout the product life cycle: design, manufacturing, testing, certification, training, maintenance, and operations. Reduced Order Modeling (ROM) using machine learning is improving the fidelity of modeling and simulation (M&S). However, Digital Twins are not a substitute to operational testing and evaluation (OT&E) in the real world physical environment. In the aviation domain for example, physical flight testing is necessary for regulatory certification to account for the inherent limitations of M&S such as coupled physics (e.g., highly non-linear dynamics during stall at high angle of attack), approximations and errors in numerical computations, unpredictable operational scenarios, and various other uncertainties.
We believe it is time to move on altogether from the “Turing Test” in AI. Alan Turing was a genius who made significant contributions to science, but his work should be put in the proper context. In safety critical domains like commercial aviation, the objective of testing an AI-enabled autonomous aircraft is certainly not to fool other pilots in the airspace into believing that a human pilot is in command.
Learning-enabled components (LEC) using machine learning introduce new testing, verification, and certification challenges resulting from difficulties in providing formal guarantees of system behavior — a concern that is exacerbated by known vulnerabilities such as adversarial attacks against Deep Neural Networks; Out of Distribution (OOD) inputs and robustness to distribution shifts; lack of uncertainty estimation and calibration; and reward hacking in Reinforcement Learning. LLMs are introducing new attack vectors such as Adversarial Prompting and Prompt Injection attacks.
Systems-Theoretic Process Analysis (STPA) is a hazard analysis method that accounts for interaction between system components including software, hardware, and human errors. Safety challenges can be addressed through Formal Methods, Verification, Validation, and Uncertainty Quantification (VVUQ), Run Time Assurance (RTA), Reachability Analysis, Fault Detection, Isolation, and Recovery (FDIR), and Dynamic Fault and Attack Injection.
We believe that a unified theory of machine intelligence is needed, and we are approaching that challenge from a complex systems theory perspective. Networks (of arbitrary computational nodes, not just neural networks), emergence, and adaptation are key relevant concepts in the theory of complex systems. In particular, intelligent behavior can emerge from the interaction between diverse learning algorithms. In their drive to survive, predators and prey co-evolve through their interaction in nature. Our current biosphere is the product of 3.8 billion years of evolution and adaptation. The body of a human contains in average between 30 and 40 trillion of cells. The ratio of the number of bacteria in the microbiome over the number of human cells is commonly estimated to be 10 to 1. The complex interaction networks between hosts (human, animal, or plant) and their microbiomes — including their role in health and disease — are forcing evolutionary biologists to reconsider our notion of the individual or “self”. This includes the interaction between the gut microbiome and the brain in humans. In a paper titled A Symbiotic View of Life: We Have Never Been Individuals, Gilbert et al. wrote:
“Thus, animals can no longer be considered individuals in any sense of classical biology: anatomical, developmental, physiological, immunological, genetic, or evolutionary. Our bodies must be understood as holobionts whose anatomical, physiological, immunological, and developmental functions evolved in shared relationships of different species.”
Culture also plays an important role in both cognition and evolution.
As a practical application of the EEL framework to the aviation domain, in another post, I discuss levels of autonomy in aviation and challenges to the certification of learning-enabled systems (LES) including formal methods, explainability, uncertainty quantification, and the neuroergonomics of human-AI teaming.
The eel is a fascinating sea creature and nature is the main source of inspiration for our work at Atlantic AI Labs. Check this YouTube video to learn more about how scientists are trying to uncover the mysteries of the eel: “The shocking truth about eels”.